SBM-RGBD dataset
Updated on July 13th, 2017
The SBM-RGBD dataset has been created in order to evaluate and compare scene background modelling methods for moving object detection on RGBD videos.
It provides all facilities (data, ground truths, and evaluation scripts) for the
SBM-RGBD Challenge, organized in conjunction with the Workshop on
Background Learning for Detection and Tracking from RGBD Videos
(RGBD2017, http://rgbd2017.na.icar.cnr.it/).
The dataset and the results of the SBM-RGBD Challenge will remain available also after the competition, as reference for future methods.
Videos
Video categories
Ground truths
Performance evaluation
Download
How to cite the dataset (updated info)
Dataset organizers
Videos
The SBM-RGBD dataset provides a diverse set of groundtruthed synchronized color and depth sequences acquired by the Microsoft Kinect.
The dataset consists of 33 videos (~15000 frames) representative of typical indoor visual data captured in video surveillance and smart environment scenarios,
selected to cover a wide range of scene background modeling challenges for moving object detection.
The videos come from our personal collections as well as from the following public datasets:
- GSM dataset, described in:
G. Moyà-Alcover, A. Elgammal, A. Jaume-i-Capó, J. Varona, Modeling depth for nonparametric foreground segmentation using RGBD devices, Pattern Recognition Letters, in press, 2017
- MULTIVISION, described in
E.J. Fernandez-Sanchez, J. Diaz, E. Ros, Background Subtraction Based on Color and Depth Using Active Sensors, Sensors 13, 2013, Pages 8895-8915.
- Princeton Tracking Benchmark, described in
S. Song and J. Xiao. Tracking Revisited using RGBD Camera: Unified Benchmark and Baselines, Proceedings of 14th IEEE International Conference on Computer Vision (ICCV2013).
- RGB-D object detection dataset, described in
M. Camplani, L. Salgado, Background Foreground segmentation with RGB-D Kinect data: an efficient combination of classifiers, Journal of Visual Communication and Image Representation 25(1), 2014, Pages 122-136.
- UR Fall Detection Dataset, described in
B. Kwolek, M. Kepski, Human fall detection on embedded platform using depth maps and wireless accelerometer, Computer Methods and Programs in Biomedicine 117(3), 2014, Pages 489-501.
The videos have 640x480 spatial resolution; depths are recorded at either 16 or 8 bits. The length of the videos varies from 70 to 1400 frames.
Video categories
The RGBD videos span 7 categories, selected to include diverse scene background modelling challenges for moving object detection
related only to the RGB channels (RGB), only to the depth channel (D), or related to all the channels (RGB+D):
- Illumination Changes (RGB): Videos containing strong and mild illumination changes
(IlluminationChanges.zip).
Updated on June 9th, 2017, to fix problems with ROI and GT images of Ls_ds sequence.
If you already downloaded this category, you may want to just download the new ROI and GT images for sequence Ls_ds
here.
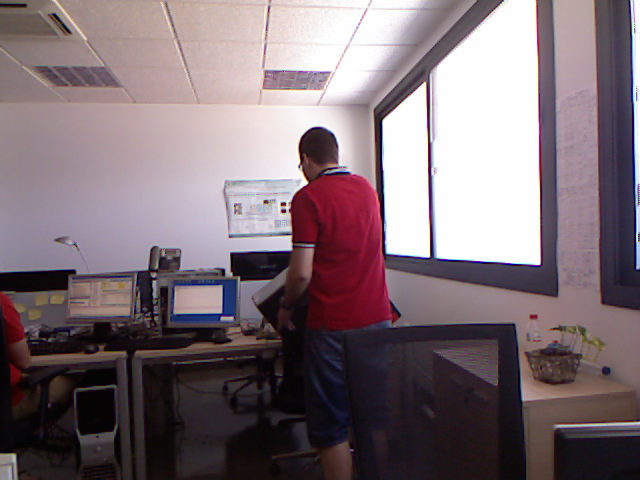 |
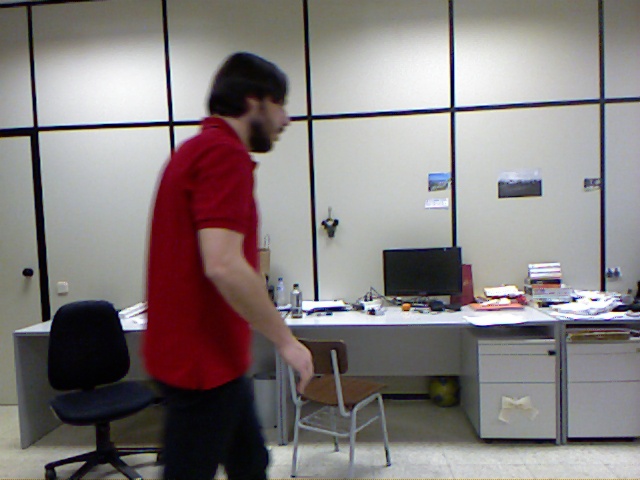 |
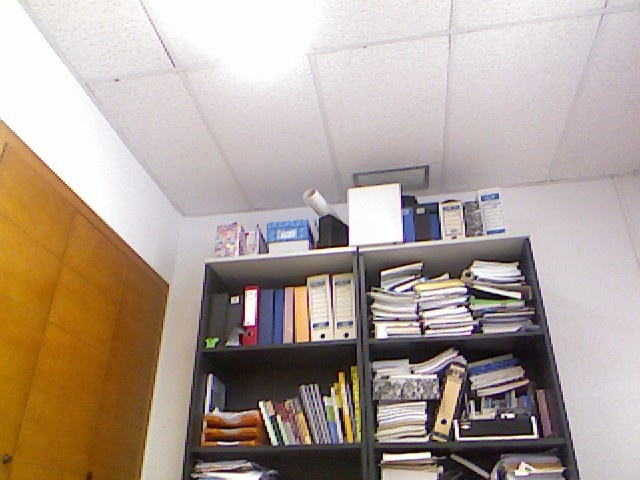 |
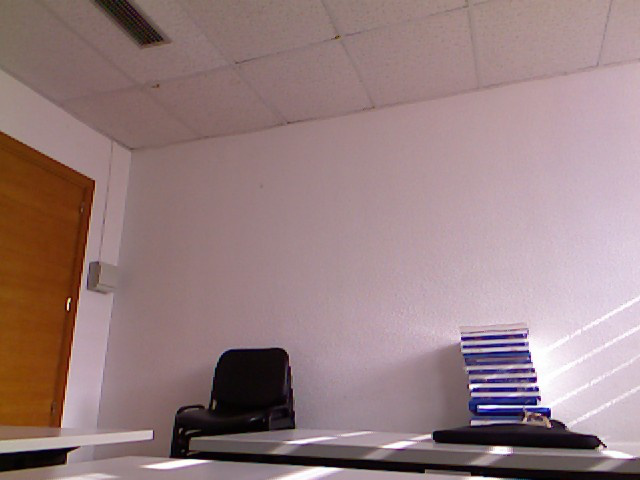 |
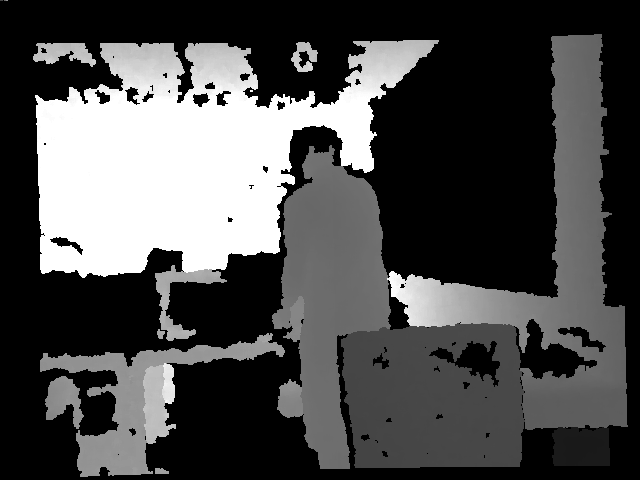 |
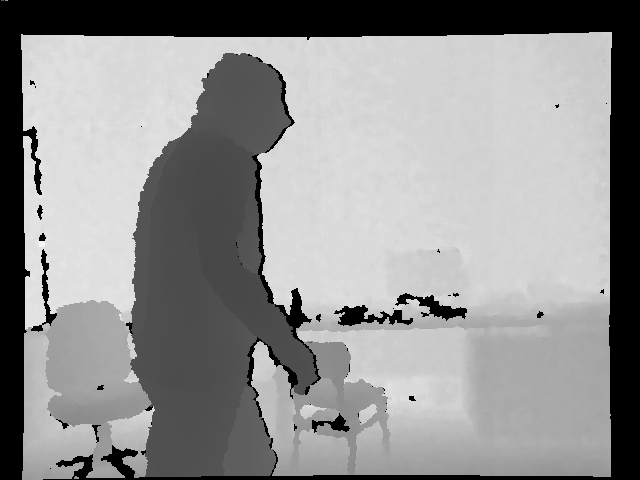 |
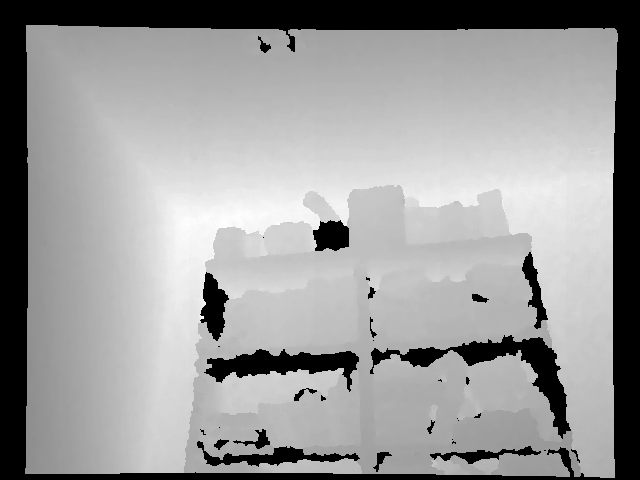 |
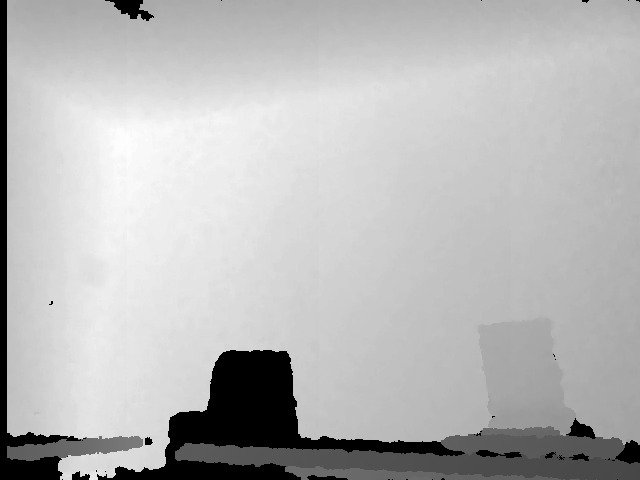 |
ChairBox |
genSeq1 |
Ls_ds |
TimeOfDay_ds |
- Color Camouflage (RGB): Videos including foreground objects that are very close in color to the background
(ColorCamouflage.zip).
Updated on June 9th, 2017.
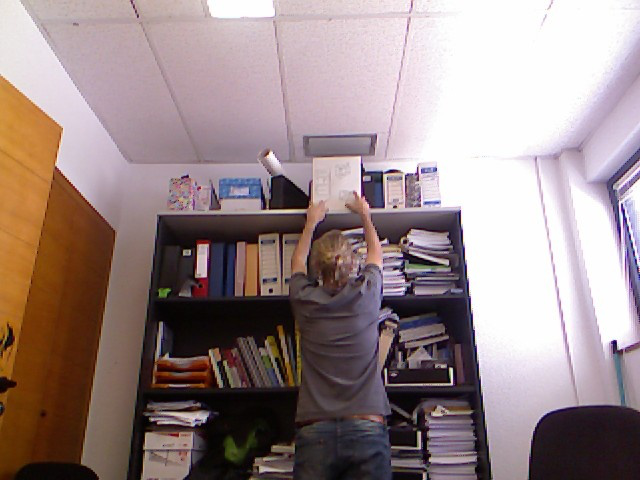 |
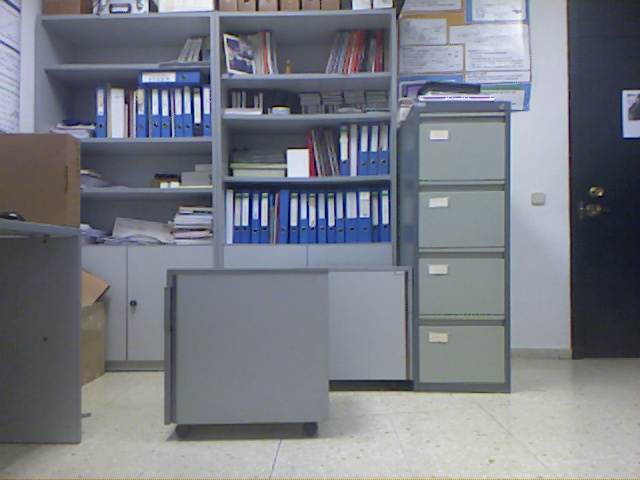 |
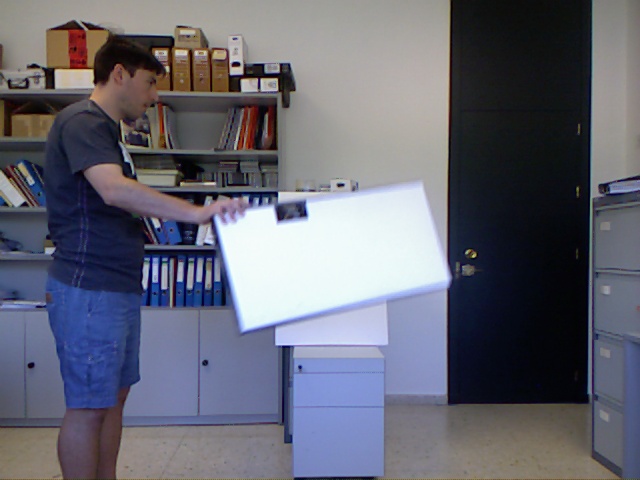 |
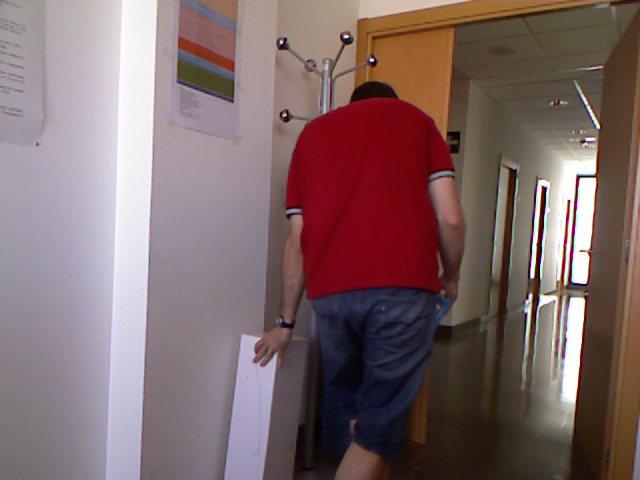 |
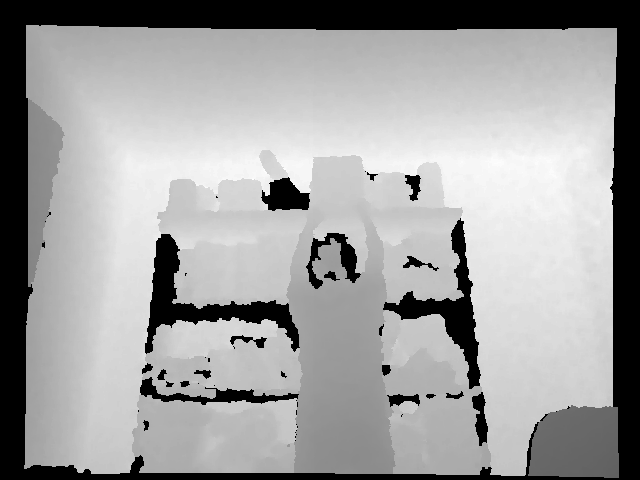 |
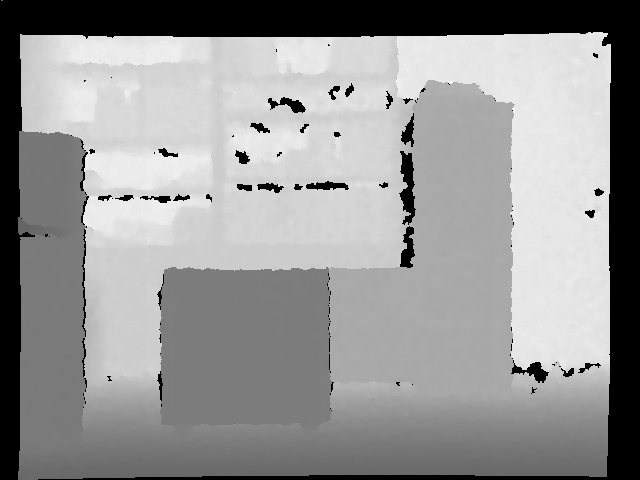 |
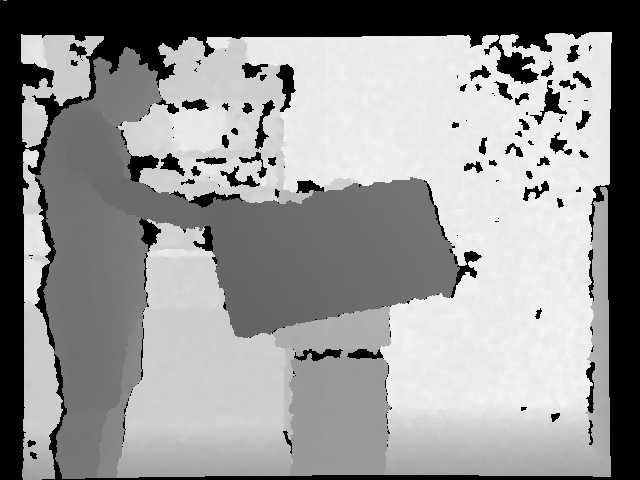 |
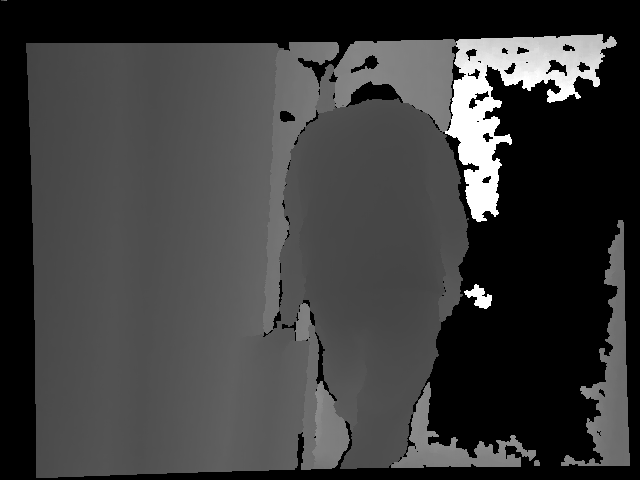 |
Cespatx_ds |
colorCam1y |
colorCam2 |
Hallway |
- Depth Camouflage (D): Videos including foreground objects very close in depth to the background
(DepthCamouflage.zip).
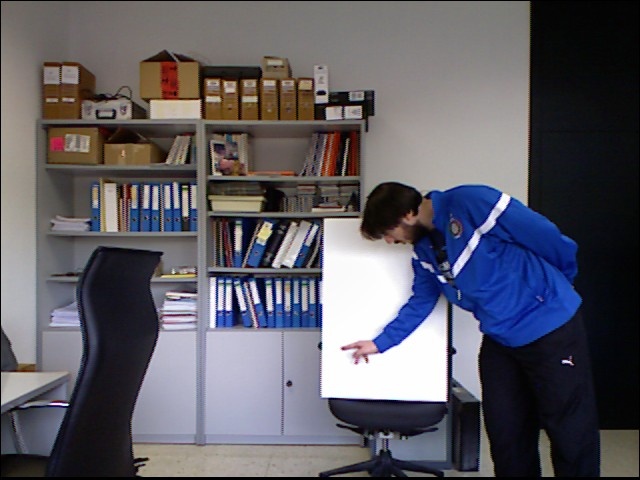 |
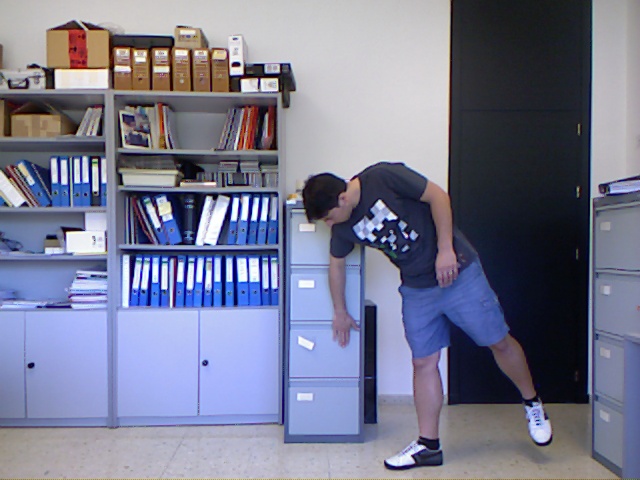 |
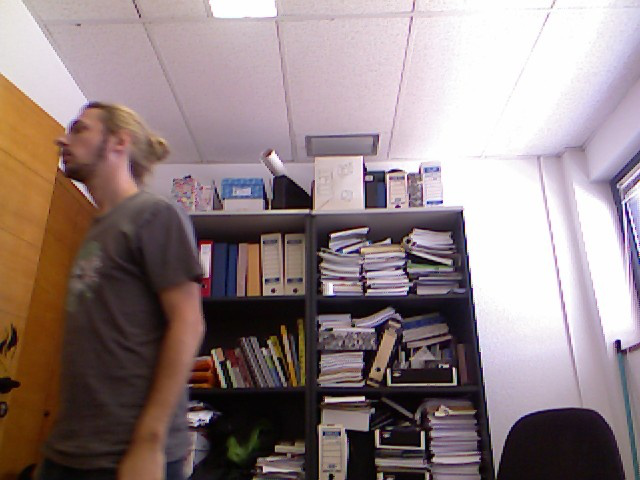 |
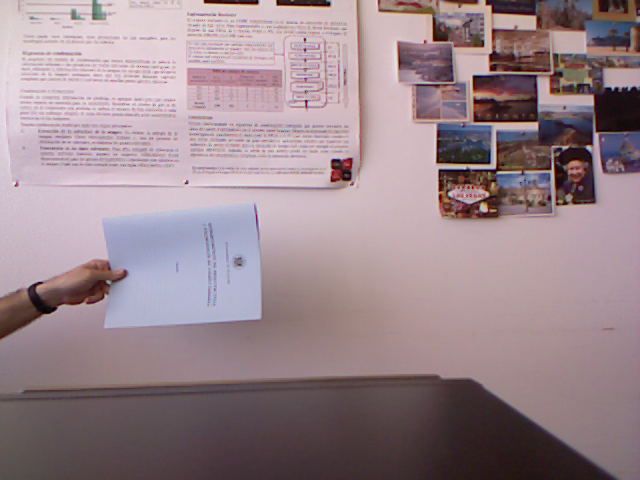 |
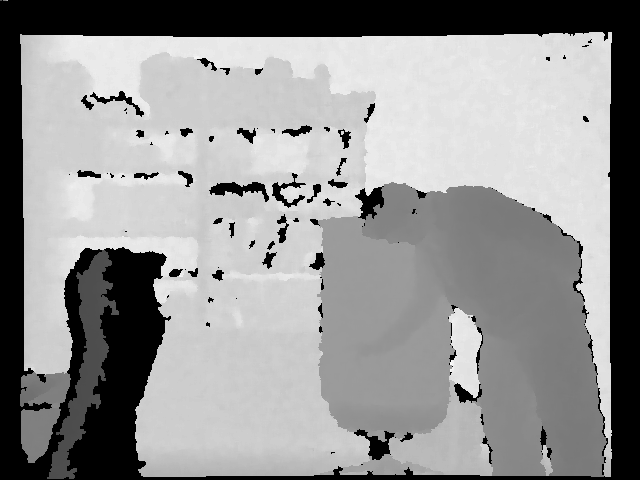 |
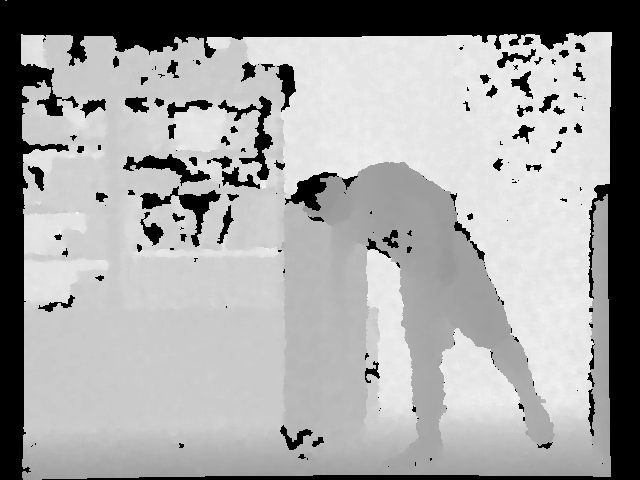 |
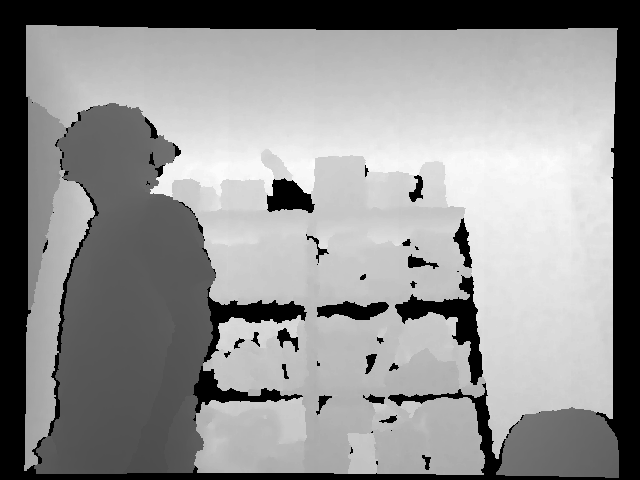 |
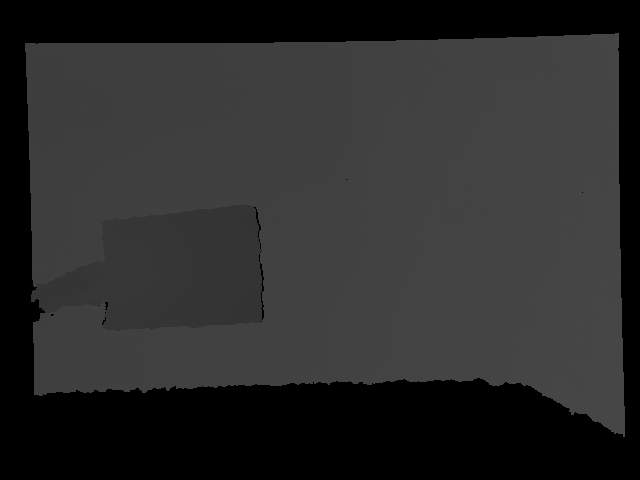 |
DCamSeq1 |
DCamSeq2 |
Despatx_ds |
Wall |
- Intermittent Motion (RGB+D): Videos with scenarios known for causing “ghosting” artifacts in the detected motion, i.e., abandoned foreground objects or removed foreground objects. Foreground objects should be detected even if they stop moving (abandoned object) or if they were initially stationary and then start moving (removed object)
(IntermittentMotion.zip).
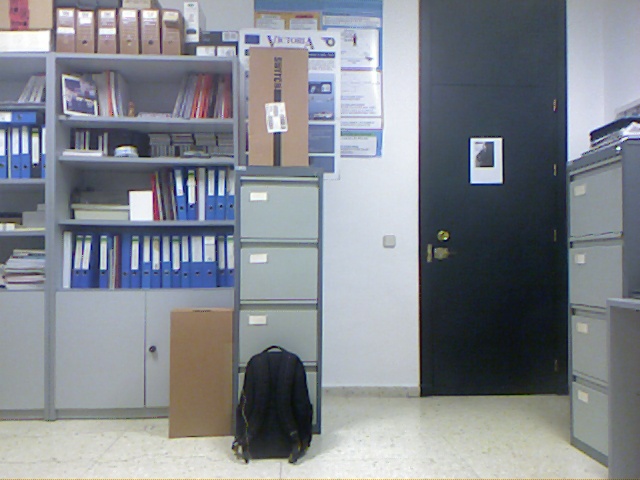 |
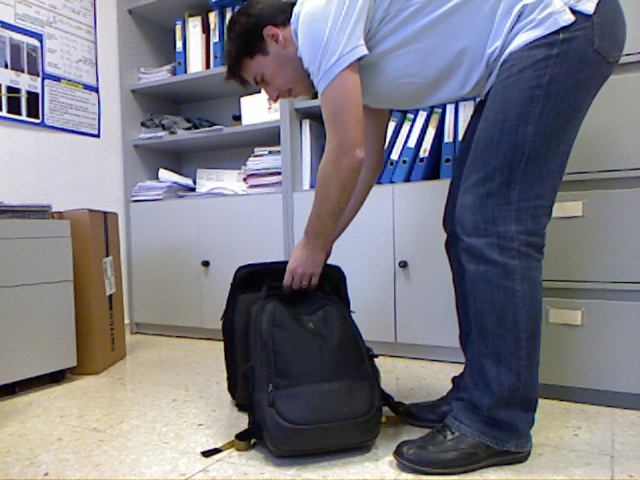 |
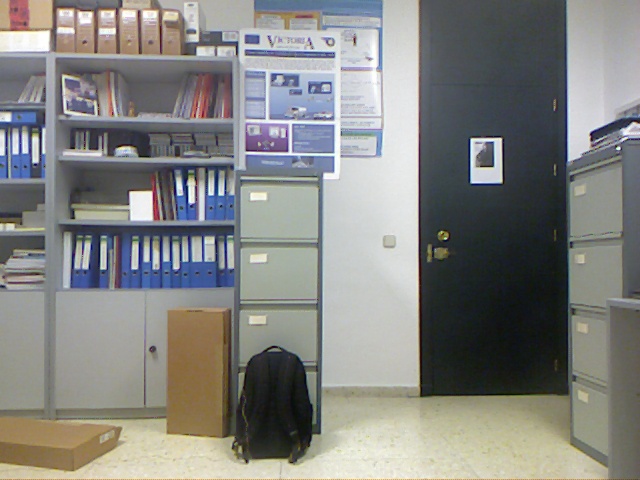 |
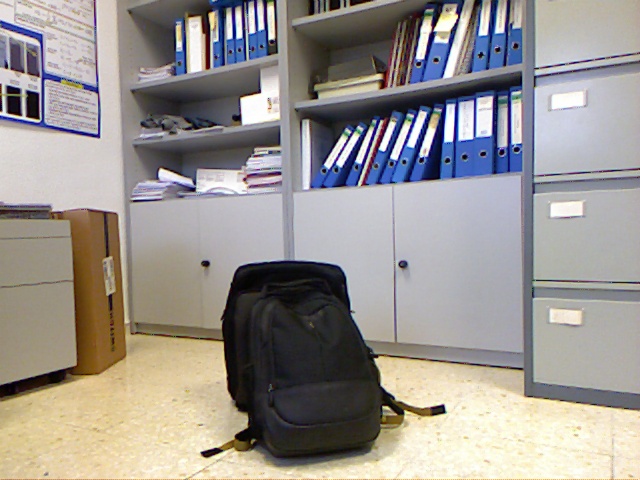 |
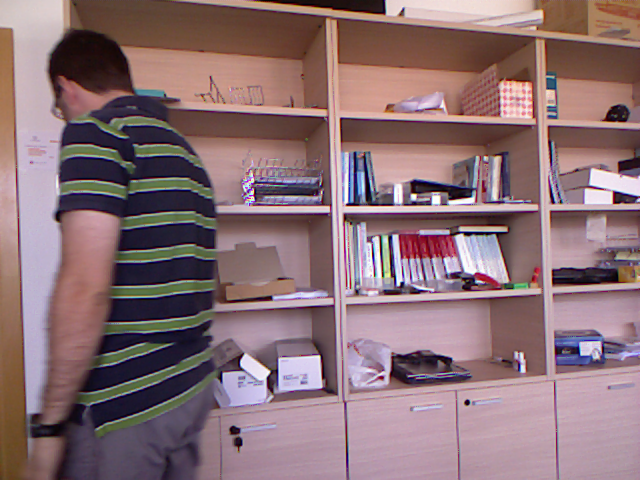 |
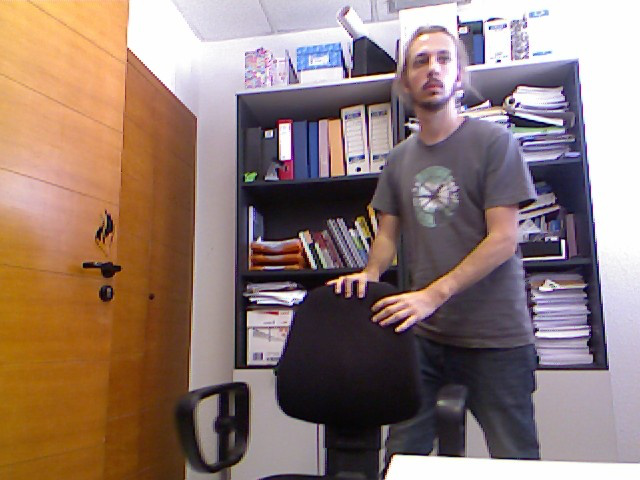 |
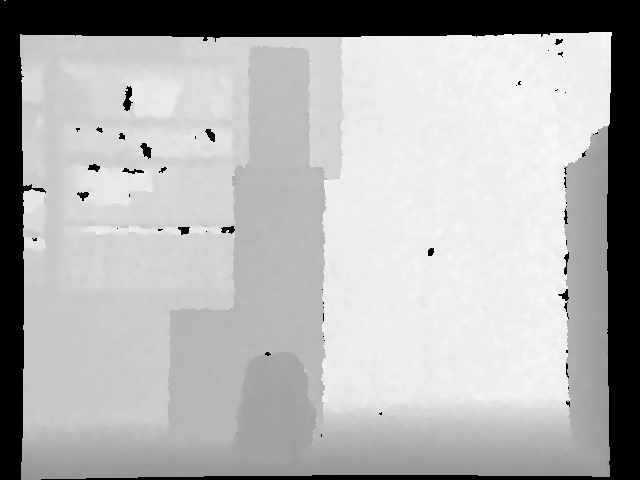 |
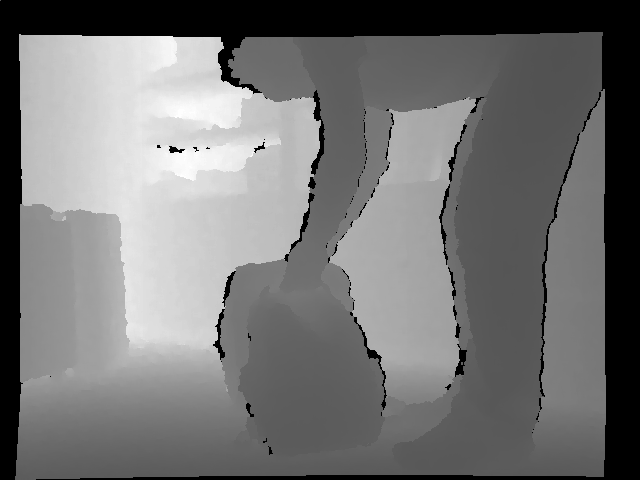 |
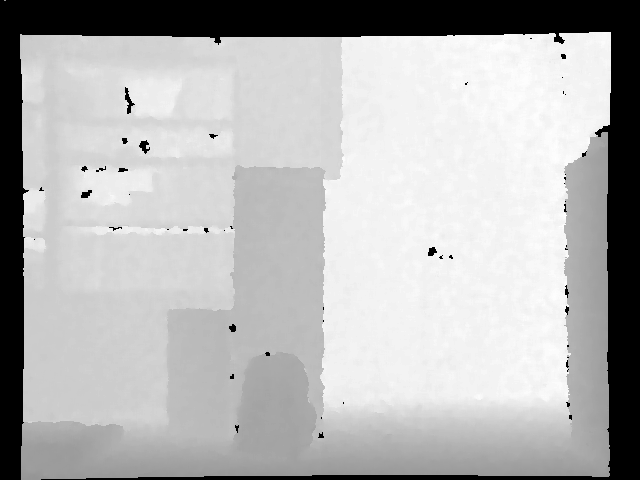 |
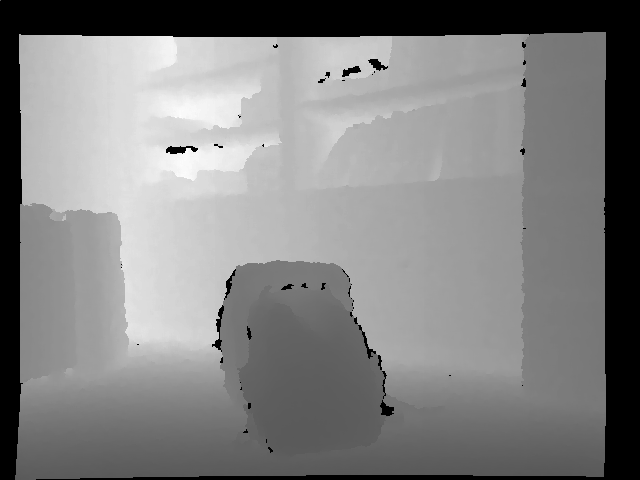 |
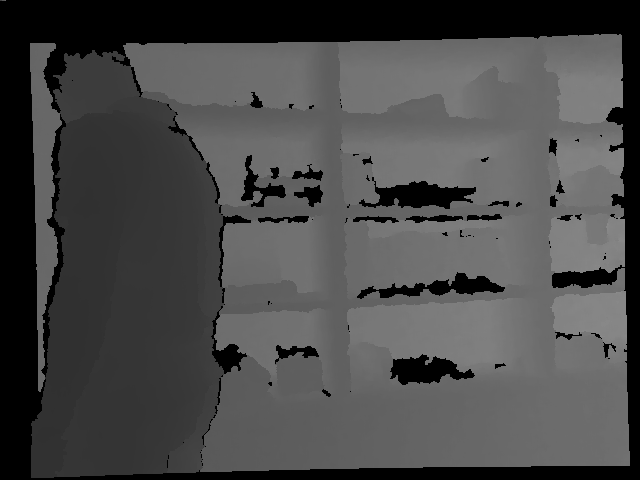 |
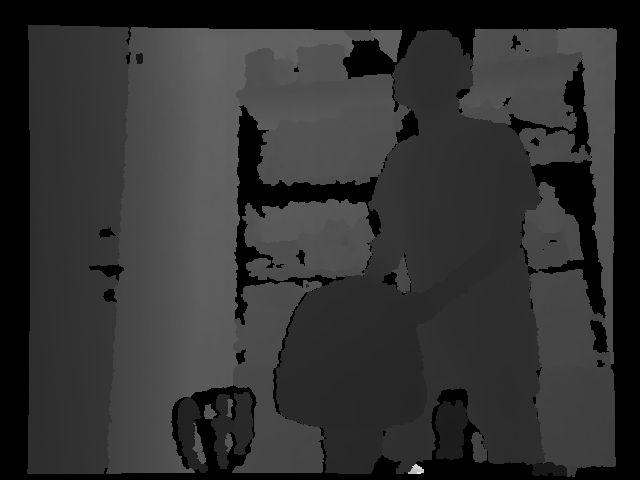 |
abandoned1 |
abandoned2 |
movedBackground1 |
movedBackground2 |
Shelves |
Sleeping_ds |
- Out of Sensor Range (D): Videos including foreground or background objects that are too close to/far from the sensor
(OutOfRange.zip). Updated on June 9th, 2017.
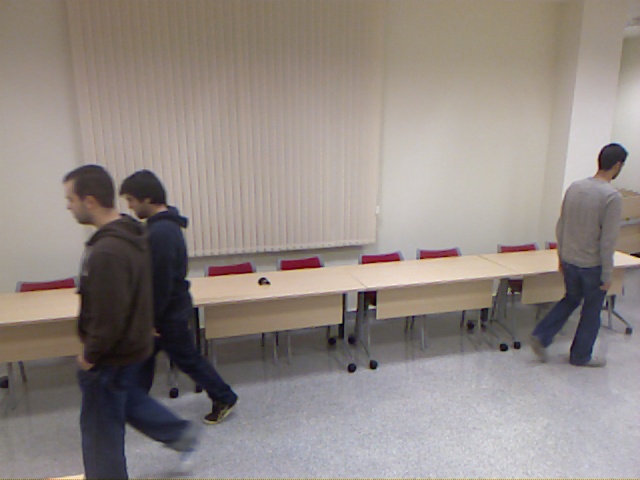 |
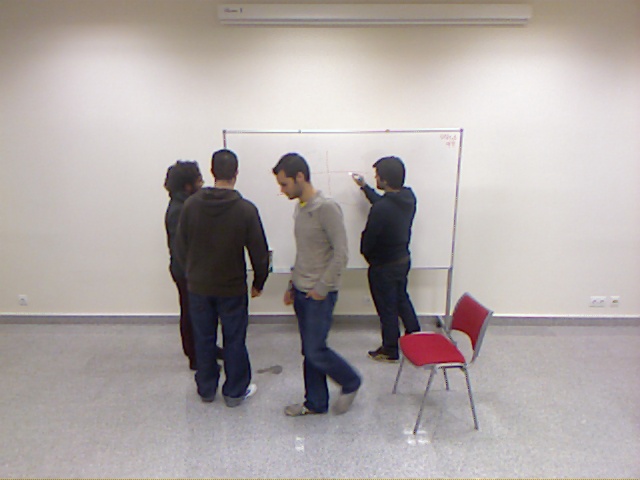 |
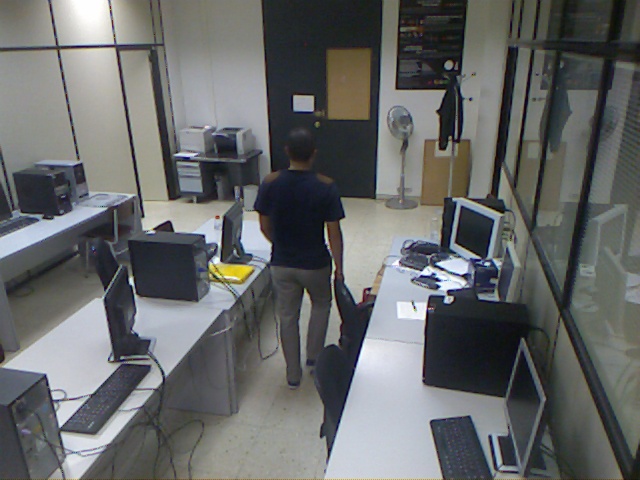 |
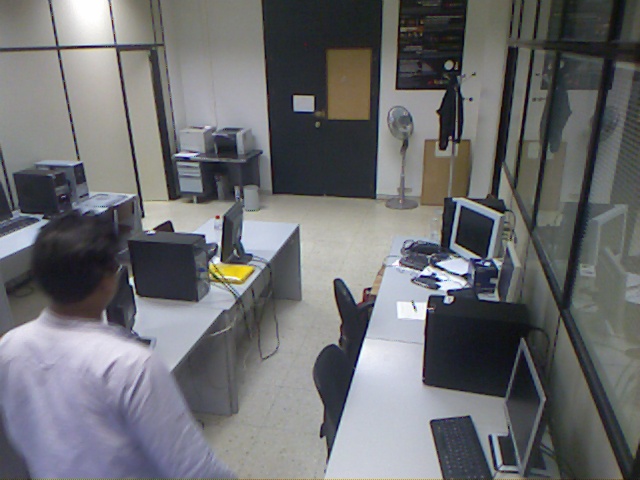 |
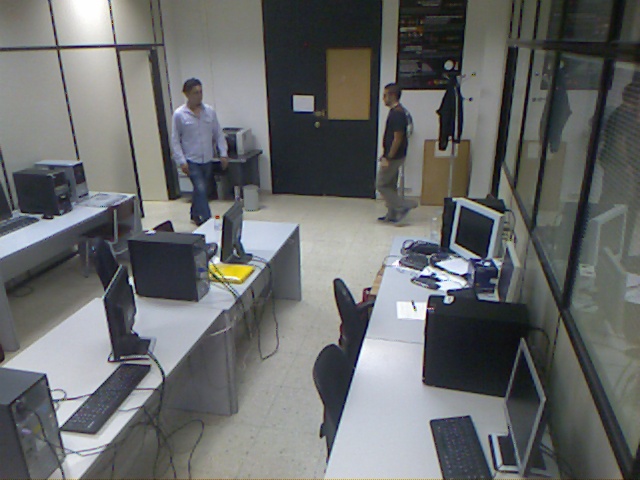 |
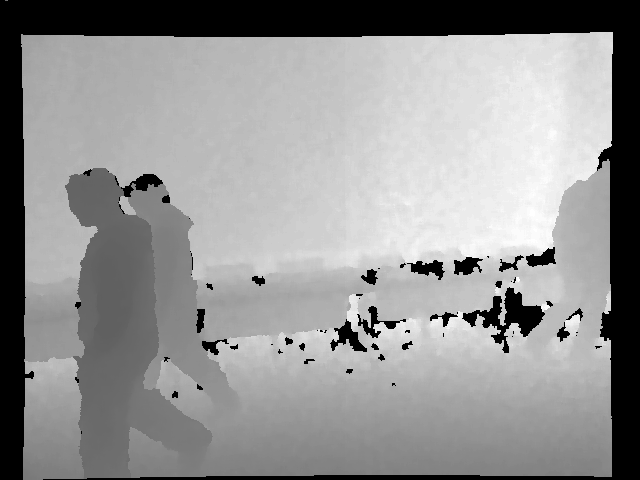 |
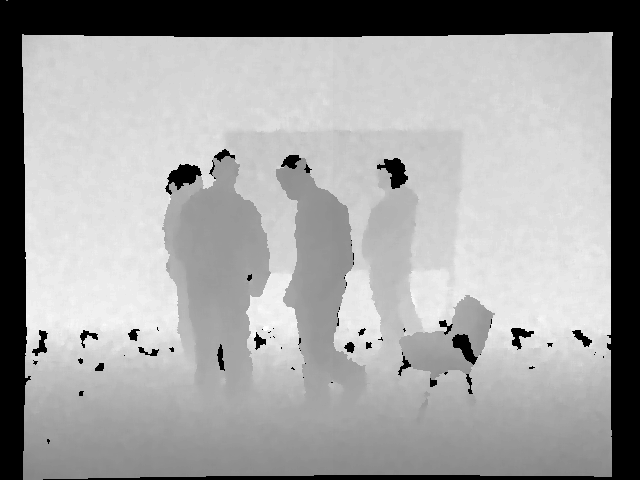 |
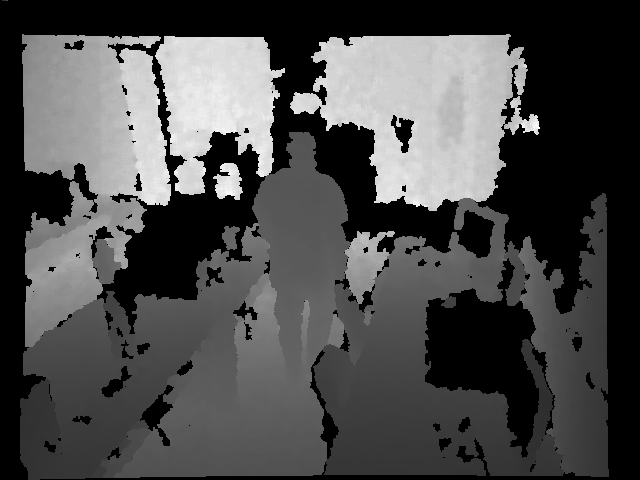 |
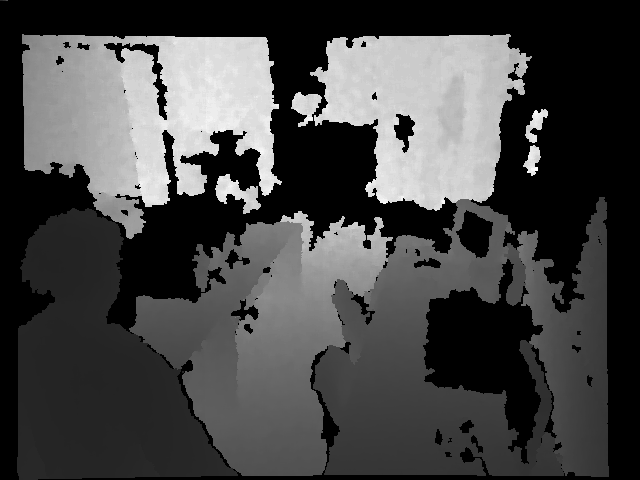 |
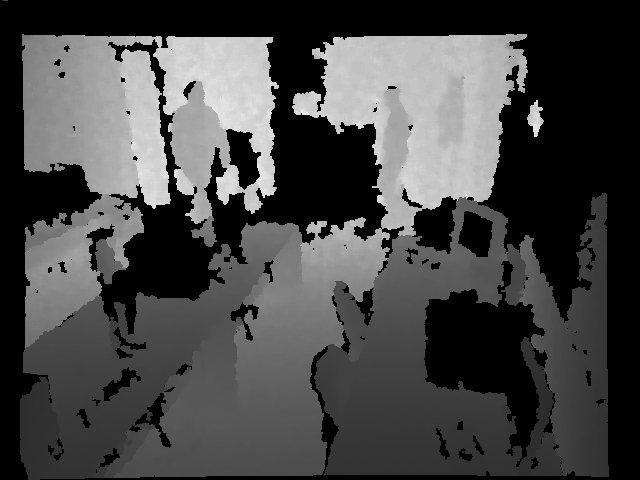 |
MultiPeople1 |
MultiPeople2 |
TopViewLab1 |
TopViewLab2 |
TopViewLab3 |
- Shadows (RGB+D): Videos showing shadows caused by foreground objects. These can be visible-light shadows in the RGB channels or IR shadows in the depth channel
(Shadows.zip). Updated on June 16th, 2017,
to scale and register depth images of fall01cam1 sequence.
If you already downloaded this category, you may want to just download the new fall01cam1 depth images, GT's and ROI
here.
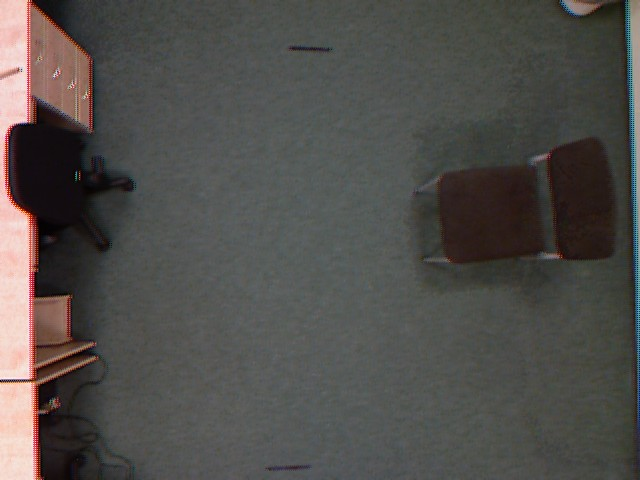 |
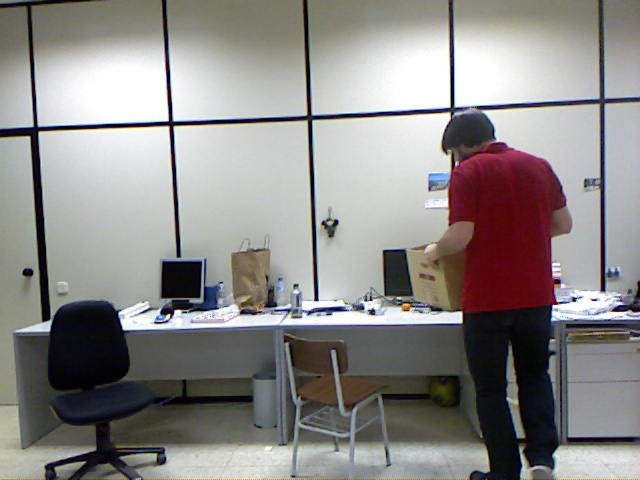 |
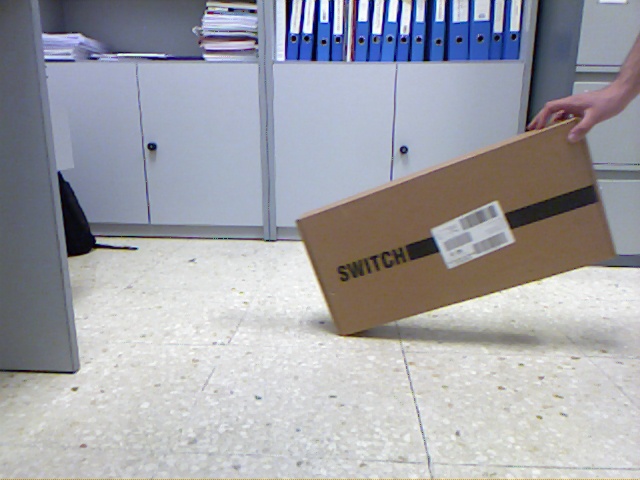 |
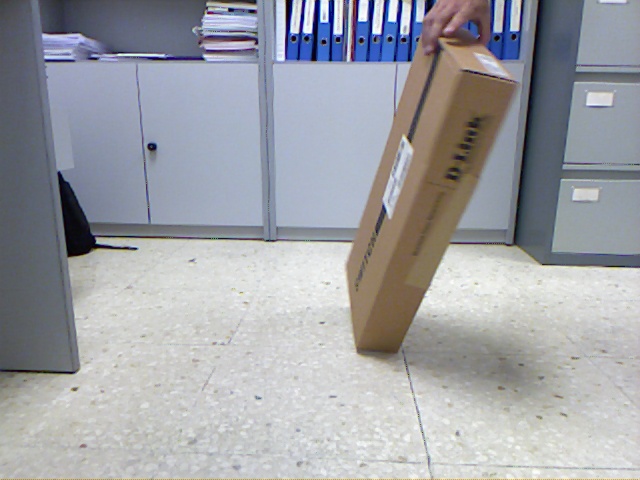 |
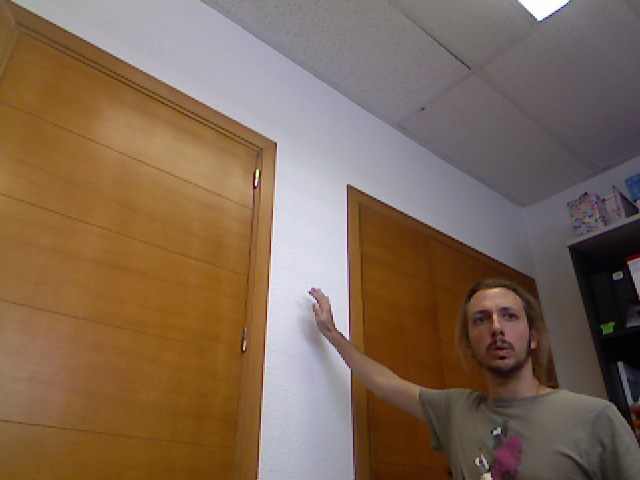 |
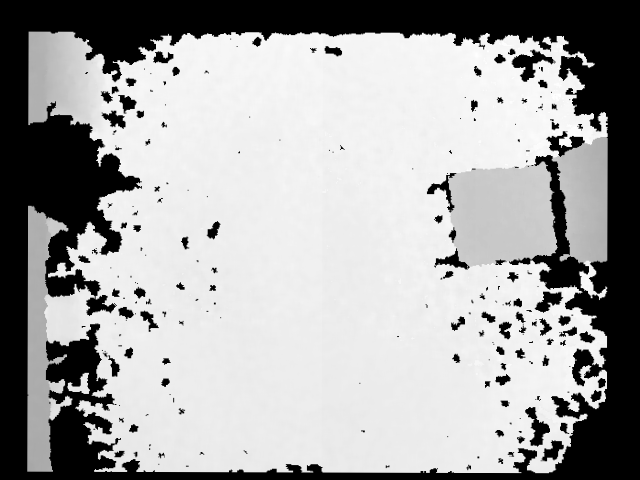 |
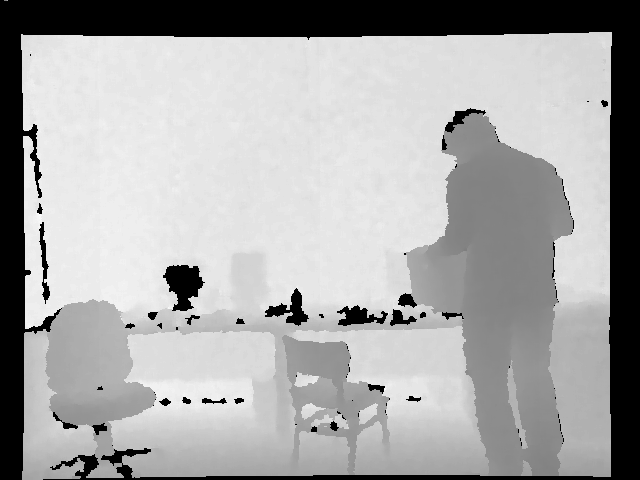 |
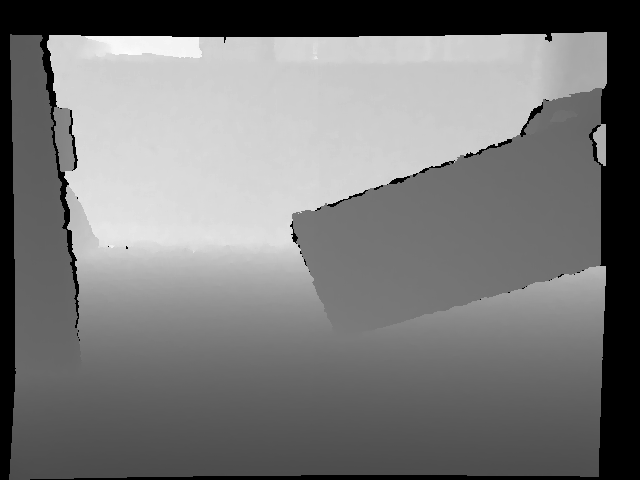 |
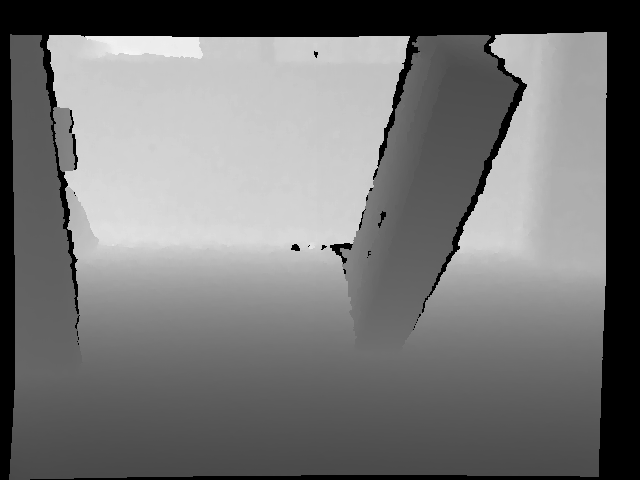 |
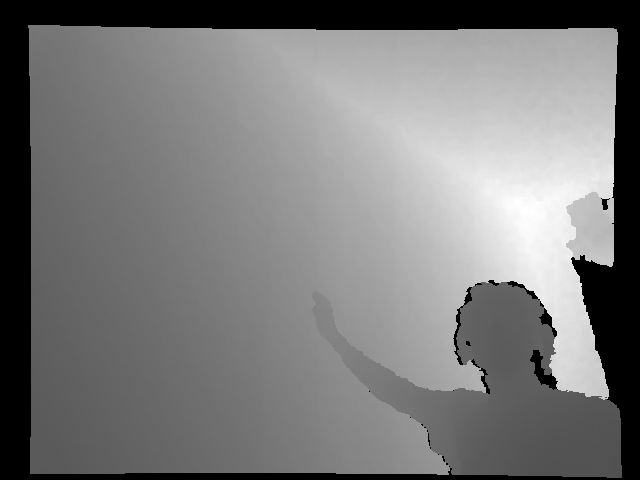 |
fall01cam1 |
genSeq2 |
shadows1 |
shadows2 |
Shadows_ds |
- Bootstrapping (RGB+D): Videos including foreground objects in all their frames
(Bootstrapping.zip). Updated on June 16th, 2017,
to scale and register depth images of adl24cam0, fall01cam0, and fall20cam0 sequences and to fix problems with some ROI and GT images.
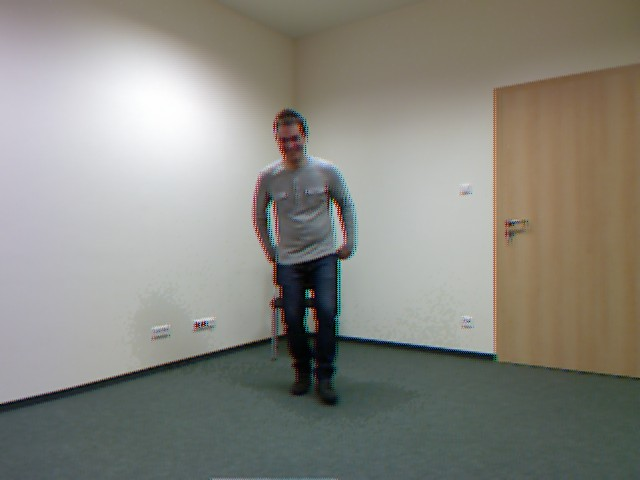 |
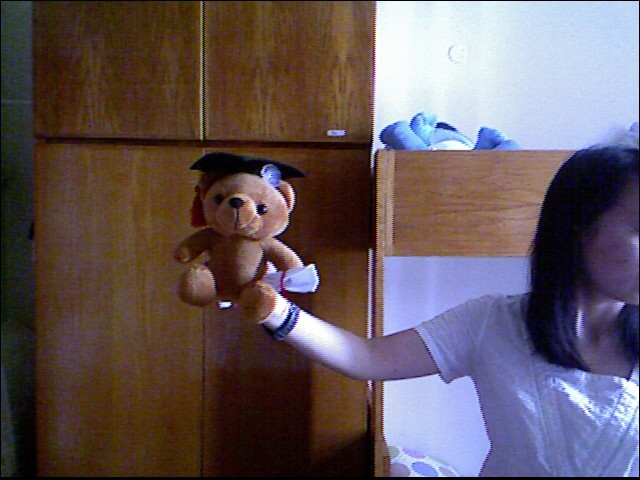 |
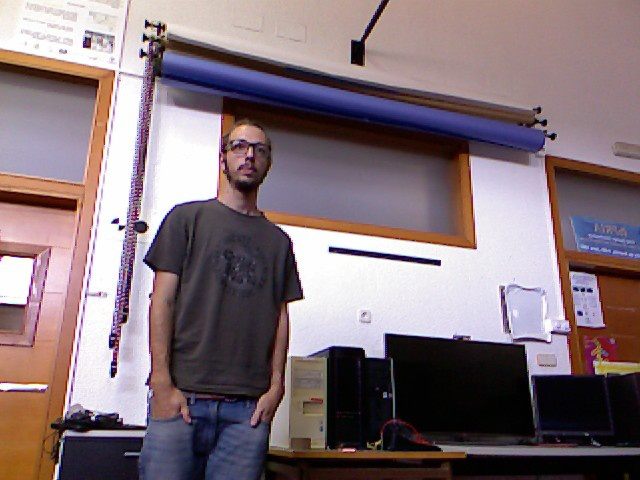 |
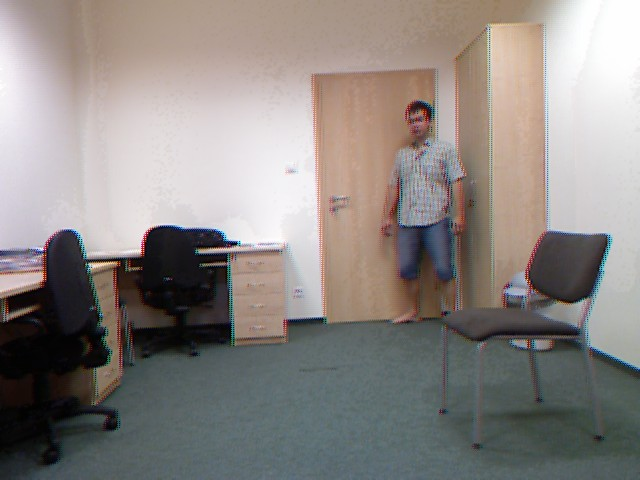 |
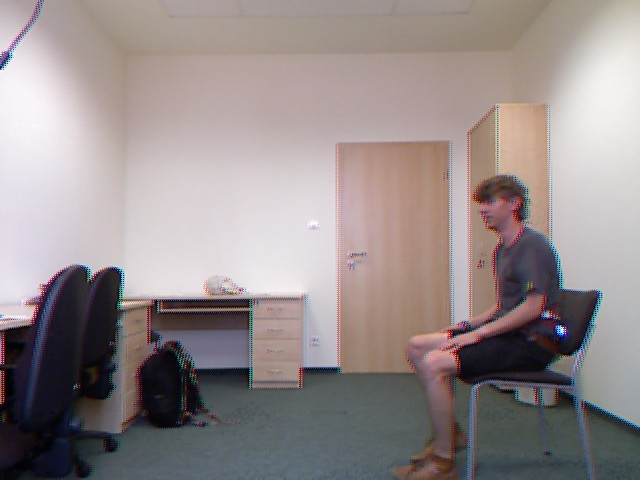 |
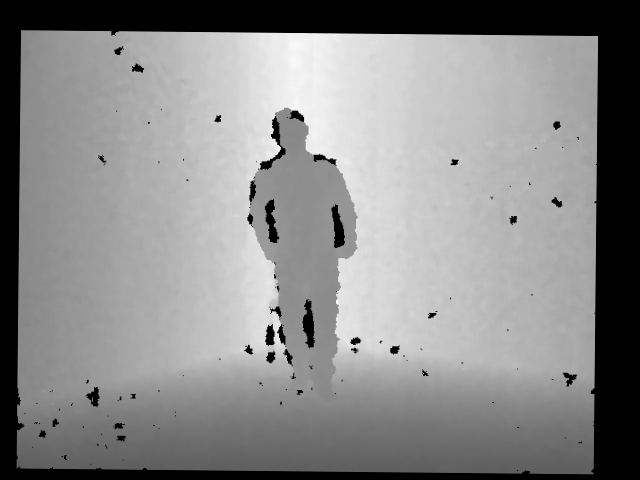 |
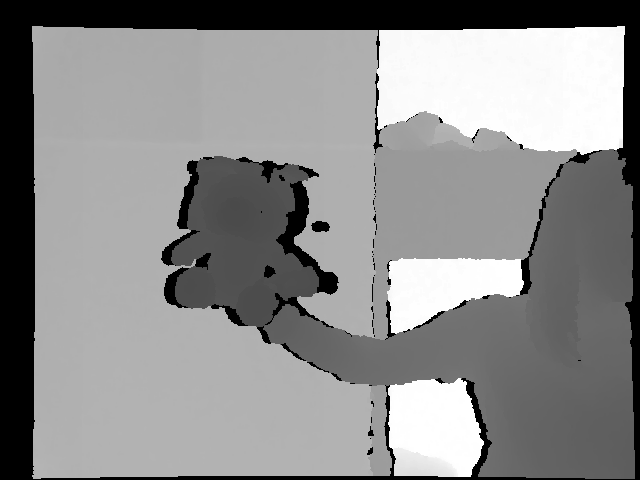 |
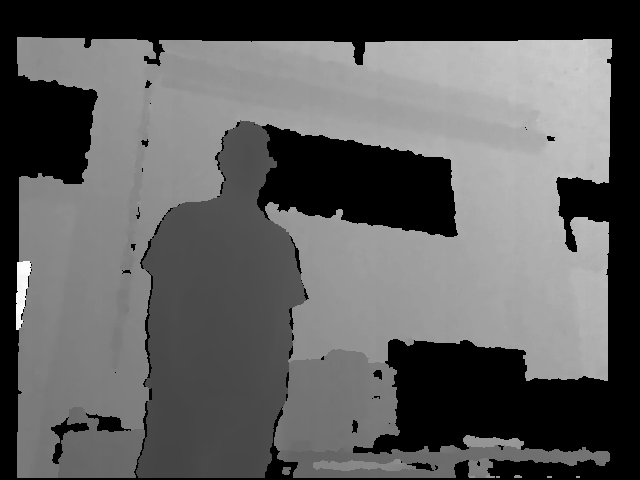 |
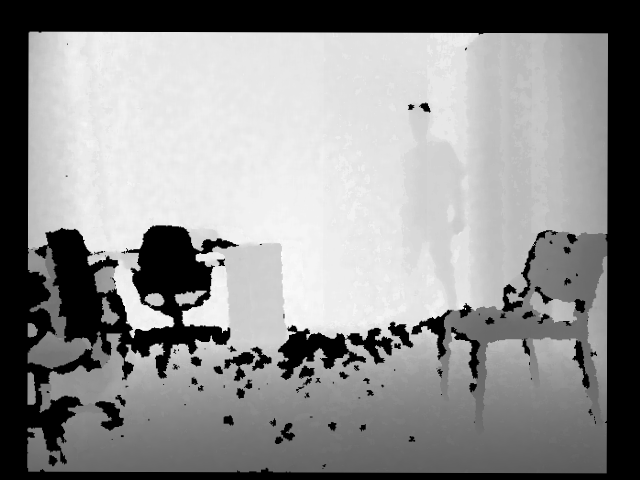 |
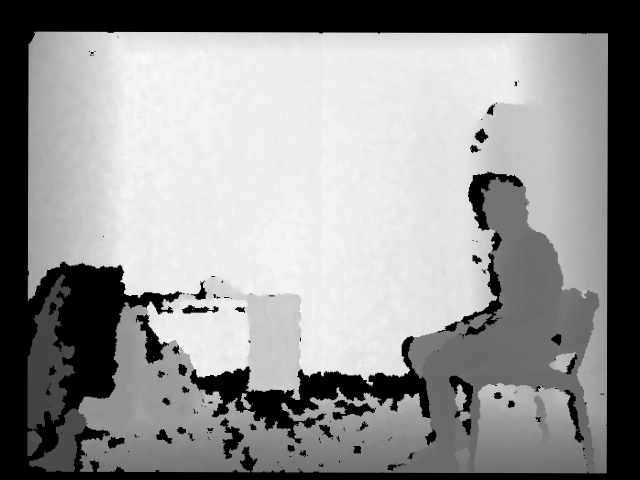 |
adl24cam0 |
bear_front |
Bootstrapping_ds |
fall01cam0 |
fall20cam0 |
Details on each video are available
here.
Ground truths
To enable a precise quantitative comparison and ranking of various algorithms for moving object detection from RGBD videos,
all the videos come with pixel-wise ground truth foreground segmentations for each video.
A foreground region is intended as anything that does not belong to the background, including abandoned objects and still persons,
but excluding light reflections, shadows, etc..
The ground truth images, some of which created using the GroundTruther software kindly made available by the organizers of
changedetection.net, contain 4 labels, namely:
- 0: Background
- 85: Outside region of interest
- 170: Unknown motion (around moving objects, due to semi-transparency and motion blur)
- 255: Foreground
Only a subset of the available ground truths is made publicly available for testing. However, for the SBM-RGBD Challenge, the evaluation is made across all the ground truths for all the videos. We hope this will reduce the possibility of overtuning method parameters.
Performance evaluation
The SBM-RGBD dataset comes also with tools to compute performance metrics for moving object detection from RGBD videos, and thus identify algorithms that are robust across various challenges.
The Matlab scripts to compute all performance metrics, provided in
MatlabScripts.zip, have been adapted by the scripts available from
changedetection.net. The metrics include
- Recall: Re=TP / (TP + FN)
- Specificity: Sp=TN / (TN + FP)
- False Positive Rate: FPR=FP / (FP + TN)
- False Negative Rate: FNR=FN / (TP + FN)
- Percentage of Wrong Classifications: PWC=100 * (FN + FP) / (TP + FN + FP + TN)
- Precision: Precision=TP / (TP + FP)
- F-Measure: F-measure=(2 * Precision * Recall) / (Precision + Recall)
where TP, FP, FN, and TN indicate True Positive, False Positive, False Negative, and True Negative pixels, respectively.
Download
All video files within one category can be downloaded as a single .zip file. Each video file, when uncompressed, becomes a directory which contains the following:
- a sub-directory named "input" containing a separate .png file for each frame of the input video.
- a sub-directory named "groundtruth" containing a ground truth mask .png file for some of the video frames.
- a file named "ROI.bmp" showing the spatial region of interest.
- a file named "temporalROI.txt" containing two frame numbers. Only the frames in this range are used to evaluate the results submitted to the SBM-RGBD Challenge.
How to cite the dataset
If you use the SBM-RGBD dataset to test and report results in any publication, we request that you acknowledge the dataset webpage
http://rgbd2017.na.icar.cnr.it/SBM-RGBDdataset.html and the following paper:
M. Camplani, L. Maddalena, G. Moyà Alcover, A. Petrosino, L. Salgado, A Benchmarking Framework for Background Subtraction in RGBD videos, in S. Battiato, G. Gallo, G.M. Farinella, M. Leo (Eds),
New Trends in Image Analysis and Processing-ICIAP 2017 Workshops, Lecture Notes in Computer Science, Springer, 2017
Dataset organizers
Massimo Camplani, University of Bristol, UK
Lucia Maddalena, National Research Council, Italy
Gabriel Moyà Alcover, Universitat de les Illes Balears, Spain
Alfredo Petrosino, University of Naples Parthenope, Italy
Luis Salgado, Universidad Politécnica de Madrid & Universidad Autónoma de Madrid, Spain